“Process optimization” as a term means different things to different people. In the context of the energy transition, it is taking on new meaning, demanding that software not only improve productivity but also balance its carbon footprint. AI is the natural candidate to deliver this double bottom line, but still faces critical issues:
- AI only works in narrow use cases where lots of data is available
- Installing sensors on all key equipment is prohibitively expensive
- Many critical industrial problems don’t have the appropriate data to justify using AI
Traditional AI has been successful in predictive maintenance for certain asset types. But there are more impactful ways that AI can influence the top and bottom lines. That next step is true process and equipment optimization, which enables higher productivity while minimizing carbon emissions.
Process Optimization with Physics and Machine Learning
Today’s AI can perform well in use cases like anomaly detection, where there is enough data to identify irregular behavior. In certain well-behaved scenarios, it’s even possible to classify the potential reasons for this behavior. In this case, the core function of AI is to predict; given a new data point, the model predicts a category based on training examples.
Optimization, on the other hand, requires higher precision. Instead of just classifying, we need AI that accurately and quickly scans millions or billions of scenarios to determine the best setpoints to achieve a specific outcome. Geminus’ platform maximizes the value of information sources, like physics-based simulation and measurement data, to create extremely accurate machine learning (ML) models that execute in real-time. Optimizing for both productivity and carbon emissions.
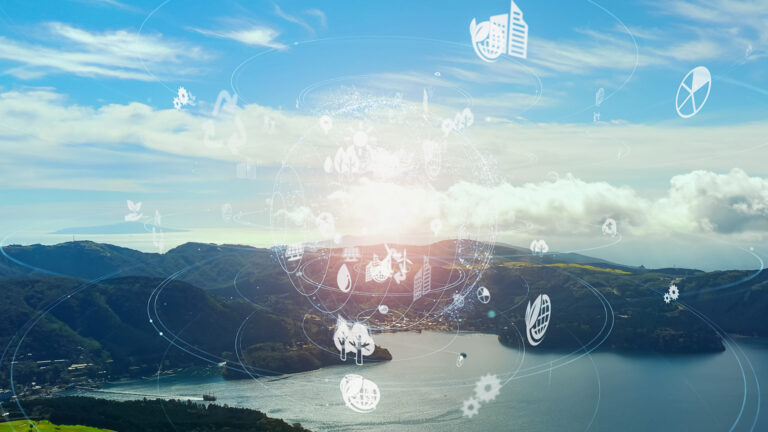
Optimization Example: Maximize Oilfield Productivity with Fixed Power
Imagine an oilfield scenario where a fixed amount of available energy must be distributed to individual electric submersible pumps (ESPs) across a network of oil wells. The goal is to allocate the power optimally across the pumps to maximize the net oil flow out of the wells. This level of insight can enable more productivity for a given carbon footprint, or a reduced carbon footprint while maintaining current production. In this case, relying on a large volume of historical process data would not provide an adequate understanding of the complex physics of a single well, let alone the flow interactions that occur in a network of tens or hundreds of wells.
The solution is to leverage physics and machine learning together. The Geminus Platform leverages existing information sources to create ML models with real-world accuracy in hours, rather than weeks. Our primary information source, in this case, is SLB’s PIPESIM, a multiphase flow simulator that captures the physics of fluid flow in the network of wells with very high accuracy. Like most simulators, the accuracy can match actual behavior extremely well with proper tuning. When coupled with Geminus, the combination can access those rigorous insights in real-time to scan all the relevant possible operational strategies and recommend the best one.
Geminus produces a model that matches the accuracy of the simulator with high inferencing speed. This model powers an intelligent advisor app that allows the user to virtually adjust power distribution to each pump in real-time and see the impact on oil flow. The app can optimize the entire network by scanning operating space and recommending the best setpoints for each ESP in just seconds.
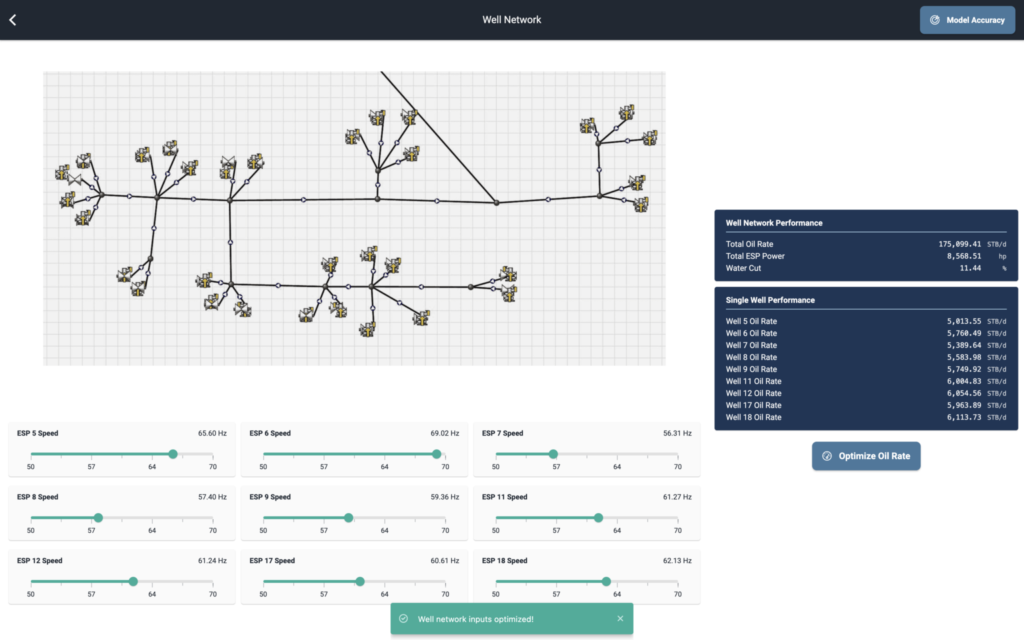
With this kind of accuracy and speed, a user can optimize for:
- Increased productivity with a fixed amount of power and emissions
- Reduced power consumption and emissions while maintaining productivity
This kind of optimization isn’t limited to well networks. There are applicable use cases for many types of plants and systems, from refineries, to power plants, to water treatment. Geminus’ multimodal approach can also leverage sensor data and analytical models as sources, in addition to sensor data and simulation, allowing for widespread industrial optimization.